Tweet Sentiment Extraction - Midway Blog
This blog is the second entry documenting my effort in the “Tweet Sentiment Extraction” kaggle competition. In this blog, we will discuss the language model to tackle this specific challenge.
The RoBERTa model
We will use the TensorFlow to construct the RoBERTa model. The model was constructed following the kaggle kernel written by Chris Deotte. Next, we show how to tokenize the text and create question answer head.
Tokenizer
We used pretrained RoBERTa Byte level Byte-pair Encoding tokenizer to convert the data into tokens. The tokenizer can be loaded by:
import tokenizers
PATH = [Path to your tokenizer files]
tokenizer = tokenizers.ByteLevelBPETokenizer(
vocab_file=PATH+'vocab-roberta-base.json',
merges_file=PATH+'merges-roberta-base.txt',
lowercase=True,
add_prefix_space=True
)
The key to find the selected text is construct a mapping between characters in the original text and the tokens transformed from the text. After tokenization, the inputs look like the below:
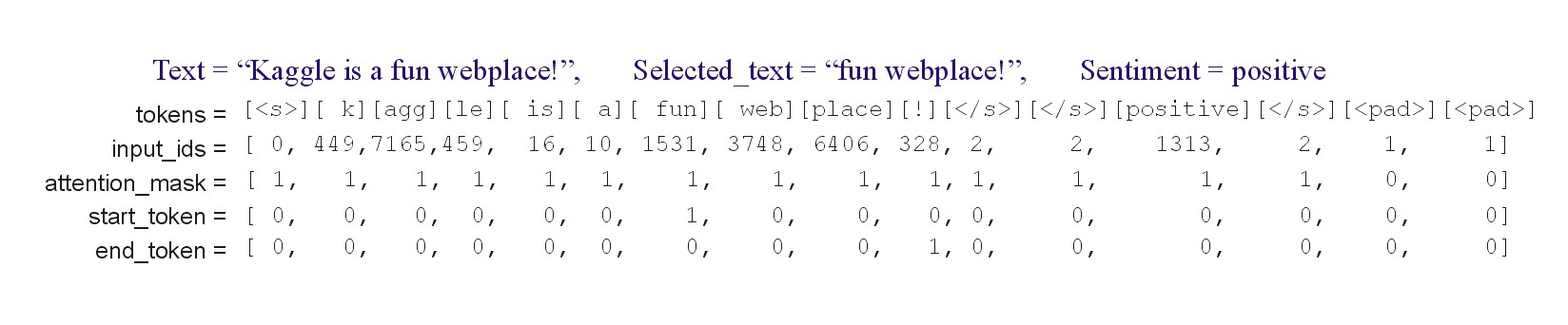
Note that the same tokenization should be applied to the test data.
Build RoBERTa model
A pretrained RoBERTa base model was used and a custom question answer head was added. First tokens were sent to a BERT model to obtain the embedding of the token sequence. The embedding went through a 1D convolution layer and activation layer to find the one-hot encodings of the start token indices. Likewise, the end index of the tokens can be found. An Adam
optimizer with a learning rate of 3e-5 and a categorical_crossentropy
were used to compile the model. The schematic diagram is shown at below:
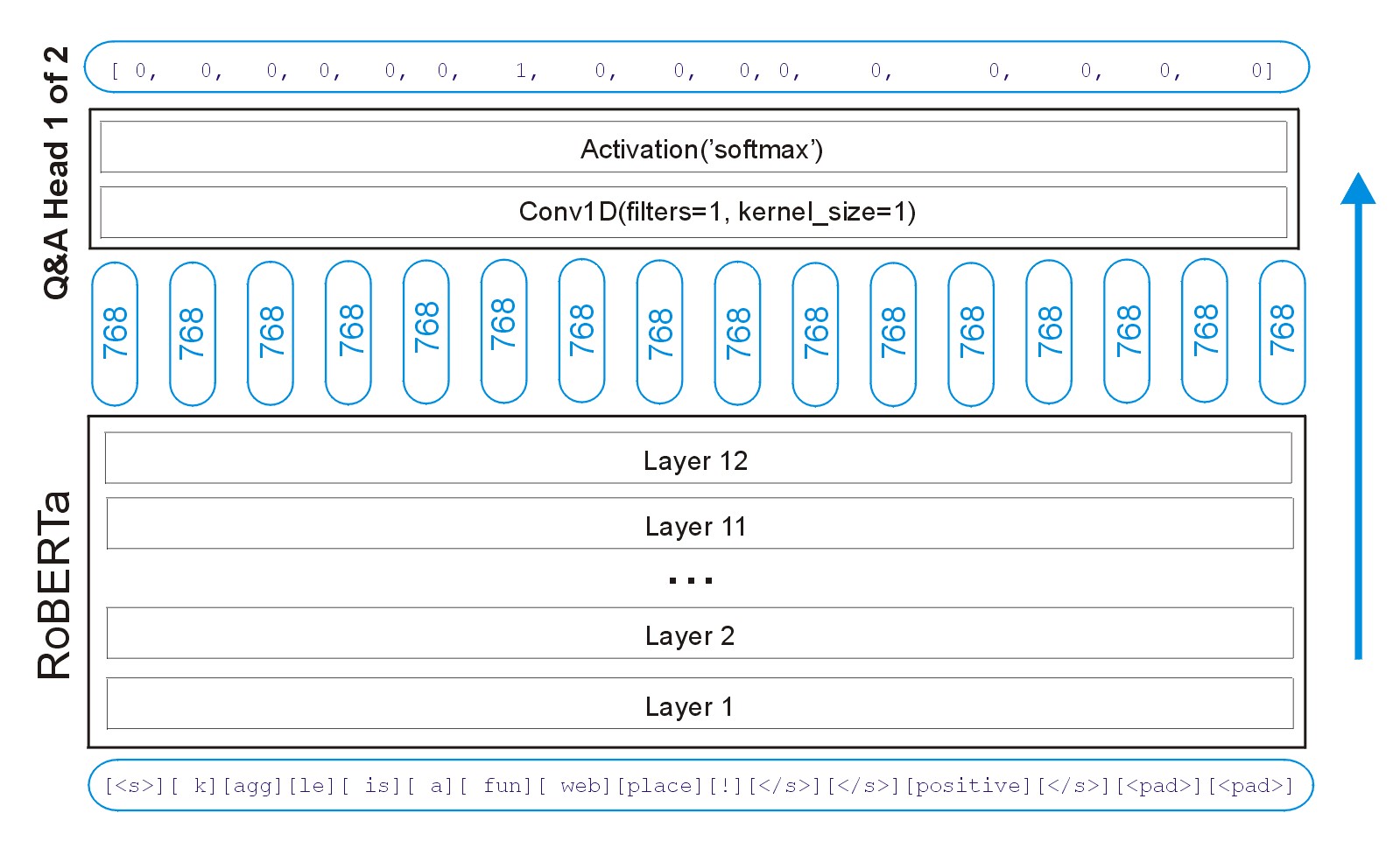
Training
The training was carried out with 5 folds stratified based on sentiment. A batch
size of 32 and 3 epochs
were used for training the model.
In order to obtain the Jaccard
score, we need to decode the identified token sequence into the text. This was achieved by:
import numpy as np
all = []
for k in idxV:
a = np.argmax(oof_start[k,])
b = np.argmax(oof_end[k,])
if a>b:
st = train.loc[k,'text']
else:
text1 = " "+" ".join(train.loc[k,'text'].split())
enc = tokenizer.encode(text1)
st = tokenizer.decode(enc.ids[a-1:b])
all.append(jaccard(st,train.loc[k,'selected_text']))
jac.append(np.mean(all))
Kaggle submission
The same decoding process should be applied to the text data. Next, we created a csv file for submission following the competition requirements. A few samples from the file looks like the below table.
test['selected_text'] = all
test[['textID','selected_text']].to_csv('submission.csv',index=False)
pd.set_option('max_colwidth', 60)
test.sample(25)
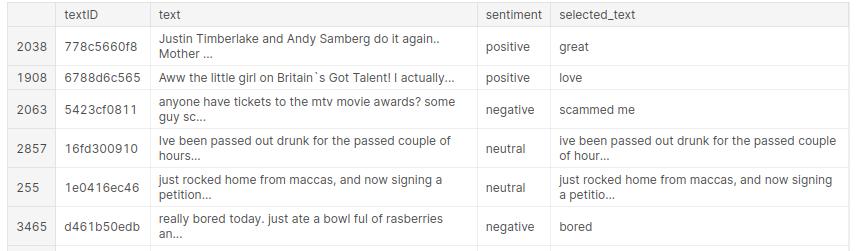