Cheng Zeng
Computational Materials Scientist at Northeastern University.
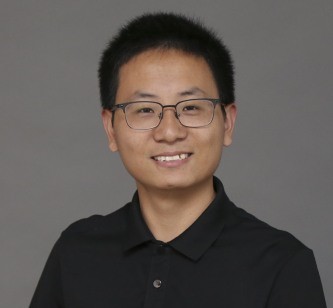
Postdoctoral Fellow
Email:c.zeng [at] northeastern [dot] edu
Hi! I am an Experiential AI postdoctoral fellow working with the Engineering team at the Roux institute of Northeastern University, co-mentored by Nathan Post and Jack Lesko. My research lies at the intersection of materials science, data science and additive manufacturing. I am interested in solving complex engineering problems with machine learning, numerical simulations and physical models. My research areas at Roux include materials design, artificial intelligence and additive manufacturing.
Prior to joining Roux, I received a PhD in Engineering at Brown University. En route to my PhD degree, I also received a secondary Master’s degree in Data Science via Brown’s Open Graduate Education Program. At Brown, I worked at the Catalyst Design Lab under the direction of Andrew Peterson. My PhD research is focused on the development of machine learning methods and atomistic physical models to accelerate rational catalyst design. A key highlight of my work is that a combination of developed machine learning and physical models enables pinpointing nanoparticle alloys with the best possible electrochemical catalysis (e.g. Co–Pt nanoparticles for oxygen reduction reaction). Prior to Brown, I got a Bachelor and Master in Materials Science and Engineering from Tsinghua University. At Tsinghua, I worked with Yunhan Ling on oxide films resistant to hydrogen permeation.
Currently I live in Portland of Maine with my wife and son. Outside office, I like long-distance running, machine learning competitions on Kaggle, and playing Nintendo Switch games.
Recent news [archive]
2024/04 | Our full proposal “Physics and Mechanics of Brittle Particle Cold Spray via Machine Learning enabled Multiscale Simulaitons” has been submitted to NSF Advanced Manufacturing Program in response to a Dear College Letter: Funding Opportunities for Engineering Research in Artificial Intelligence. It proposes to use integrated machine learning assisted simulations and experiments to overcome fundamental challenges for brittle particle cold spray, a novel additive manufacturing technique. I led the ideation and drafting of material simulations and machine learning methods for this proposal. More details of this research can be found in projects. |
---|---|
2024/02 | I am thrilled to announce that our work “Machine learning accelerated discovery of corrosion-resistant high-entropy alloys” has been published in Computational Materials Science. |
2024/02 | I gave a virtual seminar at Worcester Polytechnic Institute (WPI) Mechanical & Materials Engineering graduate seminar on Feb 21, 2024 about “Rational Design of Nanoalloy Electrocatalysts”. |