Cheng Zeng
Computational Materials Scientist at University of Florida.
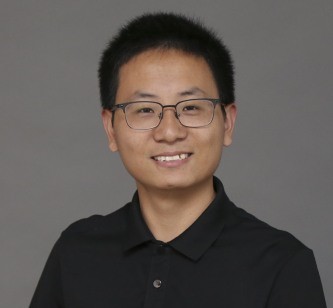
Postdoctoral Associate
Email:c.zeng@ufl.edu or cheng_zeng1@alumni.brown.edu
Hi! I am currently a postdoc working with Prof. Mingjie Liu at University of Florida. My research focuses on molecular-graph generative models and AI-driven computational study on materials for energy applications. My research interests span a multitude of topics including materials design, machine learning, electrochemistry, generative modeling, computational catalysis and renewable energy. I am interested in solving complex engineering problems with machine learning, physical simulations and numerical methods.
From 2022 to 2024, I worked at the Instutite for Experiential AI and Roux institute of Northeastern University on highly interdisciplinary topics across computational materials science, additive manufacturing, wind energy and human-data interaciton, mentored by Prof. Nathan Post and Jack Lesko. Prior to joining Roux, I received a PhD in Engineering at Brown University. En route to my PhD degree, I also received a secondary Master’s degree in Data Science via Brown’s Open Graduate Education Program. At Brown, I worked at the Catalyst Design Lab under the direction of Andrew Peterson. My PhD research focused on machine learning potentials and force–displacement phenomenological models for rational catalyst design. Before Brown, I got a Bachelor and Master in Materials Science and Engineering from Tsinghua University. At Tsinghua, I worked with Yunhan Ling on oxide films/coatings resistant to hydrogen permeation.
Recent news [archive]
2025/01 | I officially joined University of Florida Department of Chemistry as a postdoc to work on molecular-graph generative modeling and AI-driven computational simulations. |
---|---|
2024/12 | Our work “Data-efficient and interpretable inverse materials design using a disentangled variational autoencoder” is published on line at AI & Materials. We employed disentangled variational autoencoder to develop a data-efficient and interpretable inverse materials design approach, which also has the potential of design materials with multiple target properties. |
2024/09 | I am thrilled to announce that our preprint for inverse materials design using a disentangled variational autoencoder is available at arXiv. |